The rapid evolution of artificial intelligence (AI) has significantly transformed various industries, from healthcare to finance to entertainment.
One of the notable advancements in AI is the development of sophisticated language models, such as Claude 3.5, which have proven to be powerful tools in natural language processing (NLP).Claude 3.5, an AI capable of generating creative content like stories, essays, and poetry, has shown remarkable potential in Retrieval-Augmented Generation (RAG) applications.
This article explores Claude 3.5’s sonnet generation capabilities within RAG applications, detailing its technical aspects, benefits, challenges, and future directions.
Overview
Claude 3.5 is a state-of-the-art language model developed by Anthropic, designed to enhance NLP tasks through advanced contextual understanding and creative content generation. Named after Claude Shannon, the father of information theory, Claude 3.5 leverages a transformer-based architecture to process and generate human-like text across various domains.
Key Features
- Contextual Understanding: Utilizes advanced attention mechanisms to comprehend and generate coherent text based on the provided context.
- Creative Content Generation: Capable of producing diverse creative outputs, including stories, essays, poetry, and more.
- Multi-modal Capabilities: Supports the integration of text, images, and audio to deliver comprehensive and contextually rich responses.
- Customization and Fine-tuning: Allows for extensive customization to tailor the model for specific tasks or domains, enhancing its applicability.
Introduction to Retrieval-Augmented Generation (RAG)
What is RAG?
Retrieval-Augmented Generation (RAG) is an innovative approach that combines retrieval-based and generation-based methods to produce more accurate and contextually relevant text. In RAG, a retrieval component searches a large corpus of documents for relevant information, which is then used to inform the generation component in crafting a response.
Benefits of RAG
- Enhanced Contextuality: By leveraging external information sources, RAG ensures that the generated text is well-informed and contextually accurate.
- Improved Accuracy: The retrieval mechanism provides relevant data points, improving the factual accuracy of the generated content.
- Scalability: RAG can handle vast amounts of data, making it suitable for applications that require extensive knowledge bases.
Claude 3.5 in RAG Applications
Sonnet Generation
Claude 3.5’s ability to generate creative content, such as sonnets, is a testament to its advanced NLP capabilities. A sonnet, a 14-line poem with a specific rhyme scheme and meter, requires not only linguistic proficiency but also an understanding of poetic structure and themes.
How Claude 3.5 Generates Sonnets
- Input Processing: Claude 3.5 receives an initial prompt or theme for the sonnet.
- Retrieval Component: Relevant poetic phrases, themes, and structures are retrieved from a large corpus of literary works.
- Generation Component: Using the retrieved information, Claude 3.5 constructs a sonnet, adhering to the traditional sonnet structure and incorporating creative elements.
Applications of Sonnet Generation in RAG
- Literary Assistance: Assisting poets and writers in crafting sonnets and other poetic forms.
- Educational Tools: Providing students and educators with examples of sonnet structures and themes for literary studies.
- Entertainment: Creating personalized and unique poetic content for various entertainment platforms.
Technical Aspects of Claude 3.5 in RAG
Transformer Architecture
Claude 3.5 employs a transformer-based architecture, which is pivotal in handling the complexity of language generation tasks. The transformer model’s self-attention mechanism allows it to focus on different parts of the input text, capturing intricate relationships and contextual dependencies.
Attention Mechanisms
- Self-Attention: Helps the model weigh the importance of different words in the input text, enabling it to generate coherent and contextually relevant output.
- Cross-Attention: In RAG applications, cross-attention mechanisms allow the model to integrate information from the retrieved documents into the generation process, ensuring accuracy and relevance.
Fine-Tuning and Customization
Claude 3.5’s architecture supports fine-tuning on specific datasets, allowing users to customize the model for particular domains or tasks. This capability is crucial for enhancing the model’s performance in specialized applications, such as sonnet generation.
Benefits of Using Claude 3.5 in RAG Applications
Enhanced Creativity
Claude 3.5’s ability to generate creative content like sonnets showcases its potential in artistic and literary applications. By combining retrieval-based information with generation capabilities, the model produces high-quality poetic content that adheres to traditional forms.
Improved Accuracy and Relevance
The integration of retrieval mechanisms ensures that the generated content is not only creative but also accurate and relevant. This is particularly beneficial in applications that require factual correctness, such as educational tools and literary analysis.
Versatility Across Domains
Claude 3.5’s multi-modal capabilities and customization options make it a versatile tool across various domains. Whether in literature, education, or entertainment, the model’s ability to adapt to different contexts and requirements enhances its applicability.
Scalability
RAG’s ability to handle large datasets and integrate extensive knowledge bases ensures that Claude 3.5 can scale to meet the demands of various applications. This scalability is crucial for deploying the model in real-world scenarios that require processing vast amounts of information.
Challenges and Ethical Considerations
Managing Bias
Like all AI models, Claude 3.5 can be susceptible to biases present in the training data. Ensuring fairness and mitigating biases is essential to producing equitable and accurate content.
Data Privacy
In RAG applications, where external information is retrieved and integrated, maintaining data privacy and security is paramount. Implementing robust measures to protect user data and sensitive information is critical.
Ensuring Authenticity
In creative applications, such as sonnet generation, it is important to distinguish between human-generated and AI-generated content. Maintaining transparency about the origins of the content helps uphold the authenticity and integrity of artistic works.
Future Directions for Claude 3.5 in RAG Applications
Continuous Improvement
Ongoing research and development will focus on enhancing Claude 3.5’s capabilities, addressing limitations, and incorporating feedback from users. Continuous improvement ensures that the model remains at the forefront of NLP advancements.
Expanding Domain Applications
Exploring new domains and applications for Claude 3.5 will unlock its full potential. By tailoring the model to specific industries and use cases, it can provide even greater value and impact.
Integrating Advanced Retrieval Techniques
Incorporating more sophisticated retrieval techniques and expanding the knowledge base will further enhance the accuracy and relevance of the generated content. Advanced retrieval methods, such as semantic search and knowledge graph integration, can provide deeper contextual understanding.
Collaboration and Integration
Collaborating with other AI models and integrating Claude 3.5 into existing technologies will enhance its capabilities. By working with complementary models and tools, Claude 3.5 can offer more comprehensive solutions to complex problems.
Case Studies: Claude 3.5 Sonnet in RAG Applications
Literary Assistance for Poets
Claude 3.5 has been used to assist poets in crafting sonnets by providing thematic inspiration and structural guidance. The model’s ability to generate creative and contextually rich content helps poets explore new ideas and refine their work.
Educational Platforms
Educational platforms have leveraged Claude 3.5 to create interactive tools for teaching poetry. By generating examples of sonnets and explaining their structure, the model aids students in understanding and appreciating poetic forms.
Personalized Entertainment Content
In the entertainment industry, Claude 3.5 has been employed to generate personalized poetic content for users. This application showcases the model’s ability to deliver unique and engaging experiences, tailored to individual preferences.
Technical Implementation of Claude 3.5 Sonnet Generation
Data Preparation
Preparing the training data involves curating a diverse and representative corpus of poetic works. This corpus serves as the foundation for training the model, ensuring it learns the nuances of sonnet structure and themes.
Training Process
The training process involves fine-tuning Claude 3.5 on the curated corpus of poetic works. This step requires careful parameter tuning and iterative evaluation to optimize the model’s performance.
Integration with Retrieval Systems
Integrating Claude 3.5 with retrieval systems involves setting up the retrieval component to search relevant documents and extract useful information. This information is then fed into the generation component to produce contextually informed sonnets.
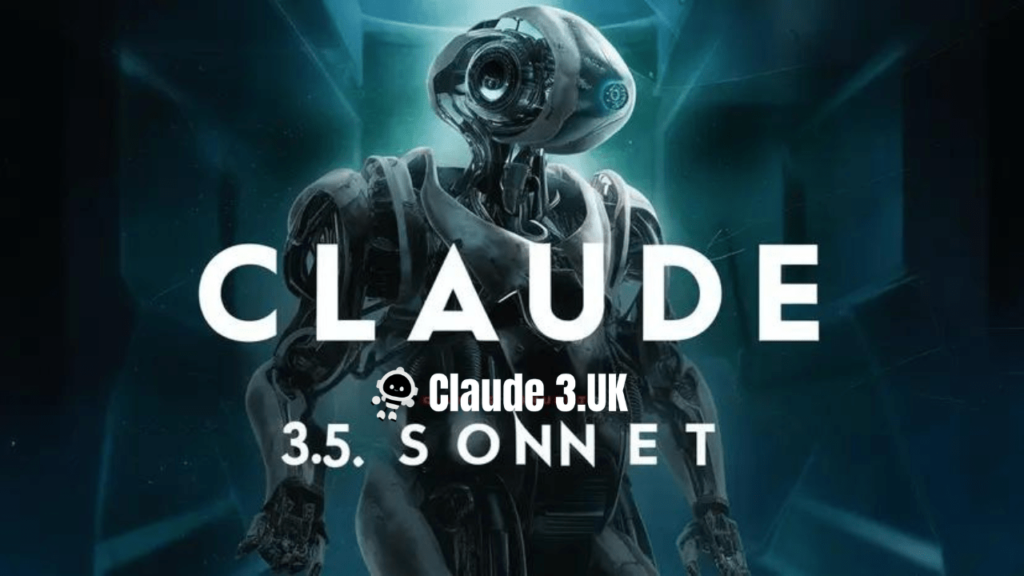
Conclusion
Claude 3.5 represents a significant advancement in the field of natural language processing, particularly in the realm of creative content generation. Its ability to generate sonnets within RAG applications highlights its potential in literary, educational, and entertainment domains.
By combining retrieval-based and generation-based methods, Claude 3.5 ensures that the generated content is both accurate and creatively rich. As research and development continue, Claude 3.5 will undoubtedly play a crucial role in shaping the future of AI-driven creativity and enhancing the capabilities of NLP applications.
FAQs
What are RAG applications?
Retrieval-Augmented Generation (RAG) applications combine retrieval-based and generation-based methods to produce text. A retrieval component searches a corpus for relevant information, which is then used by a generation component to craft accurate and contextually relevant content.
How does Claude 3.5 generate sonnets in RAG applications?
Claude 3.5 generates sonnets by first receiving an initial prompt or theme. The retrieval component searches for relevant poetic phrases, themes, and structures. Using this information, Claude 3.5 constructs a sonnet, adhering to traditional structures and incorporating creative elements.
What are the benefits of using Claude 3.5 for sonnet generation in RAG applications?
Enhanced Creativity: Produces high-quality poetic content.
Improved Accuracy: Integrates relevant information for more accurate content.
Versatility: Can be applied across various domains such as literature, education, and entertainment.
Scalability: Handles large datasets, making it suitable for extensive applications.
What future developments are expected for Claude 3.5 in RAG applications?
Continuous Improvement: Ongoing research to enhance capabilities.
Expanded Domains: Tailoring the model to new industries and use cases.
Advanced Retrieval Techniques: Integrating more sophisticated retrieval methods for deeper contextual understanding.
Collaboration and Integration: Working with other AI models and technologies for comprehensive solutions.